- June 30, 2021
- Posted by: Vaibhavi Tamizhkumaran
- Category: Data Analytics
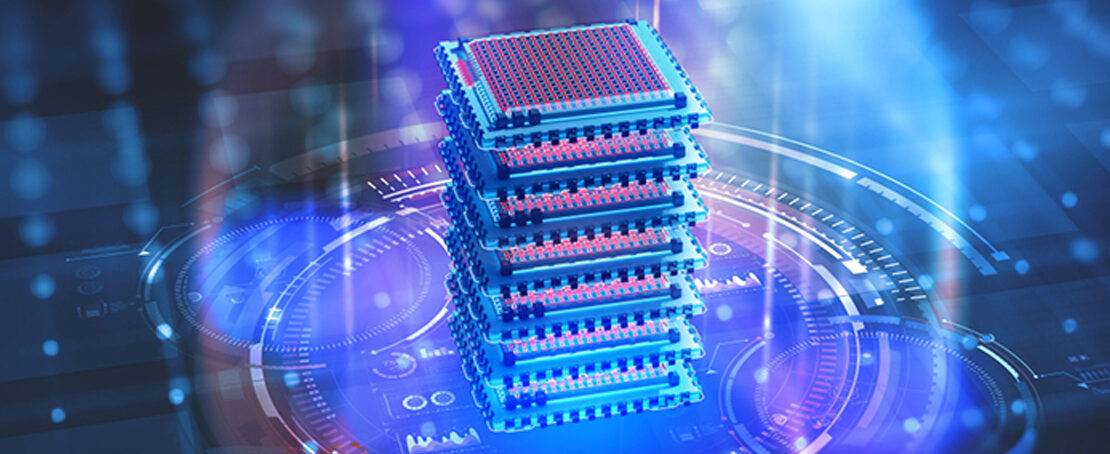
Many businesses are running to become more data-driven, increasing data usage and leveraging insights from data to improve decision-making, solving difficult problems, and increasing revenue and profitability. According to an IDC survey conducted in 2021, there is a direct relationship between quality decision-making and strong data-to-insight capability. 57% of organisations with the finest data analytics pipelines receive the highest decision-making score.
Today, against the backdrop of what can only be described as a progression of technology, the once limited and conventional field of analytics has evolved into a full-fledged industry with significant impact on the entire business ecosystem.
Data analysts are now skilled programmers and logicians. Insights, which have progressed from predictive to definitive, are now guiding business decisions at the highest levels of management. As data analytics cements its reputation as a specialty field critical to business success, the next question is how this affects the makeup of the modern data team.
Modern Data Architectures
Modern data sources are used in the design of the modern data architecture. Although traditional ETL database systems and data warehouses remain in use, the modern version of these components provide pay-as-you-use models, built-in compression, and all of the whistles we’ve come to expect from enterprise offerings, such as security, concurrency, and, most importantly, performance.
Of course, not all phases of the mortal element will be replaced on the spur of the moment by software applications. Parallel with the rise of machines as the favoured choice for regular data analytics tasks, there has been an increase in other, more specialised analytics applications that are too complex for automation – at least for the time being.
Check out our Data & Analytics Services
Read More
Infrastructure engineering is primarily concerned with the automation of data organisation frameworks.
Customized Analytics is purpose-driven and tailored, with a focus on integrating innovative technologies into the science of data analytics.
Native analysis is doubling down on better and more efficient data acquisition and management methods.
Data Transformation
The ability to transform and ingest data into the target system based on your business use case is the link between data processing and your data sources quickly and easily.
Because of the wide range of business problems that must be addressed, there is no one-size-fits-all approach to data transformation. The key is instead in the flexibility and ease of implementation of any given set of transformations, or infrastructure of transformations, to allow for more advanced model computation.
Fortunately, the data warehousing industry has recognised this, and vendors have unleashed a previously unseen set of capabilities that have proven to be game changers. Consider Snowflake, which provides a cloud-based offering that allows for the separation of compute and storage, as well as the ability to dynamically scale based on each purpose. This means that, rather than the old ETL model, the more recent ELT pattern, in which transformations can be applied on the target system itself, is faster, easier, and less expensive.
The approach you take to extracting value from your data is heavily influenced by where you fall on the business analyst to data scientist spectrum. Data analysts explore and analyse data using BI and visualisation tools. Unfortunately, the process has many manual steps: you create a theory and try slicing and dicing to try to find the key insight. The disadvantage here is obvious: how can someone find the right insight when there is so much data and so many different combinations to test?
Marketers are becoming acquainted with the fundamentals of data analysis. To develop better solutions, data engineers must now understand traditional analytics frameworks. Conventional analysts must now deal with the vastly complex business of specialty data science sub-fields.
Data scientists are now forming mini-teams with data engineers to assist in the deployment of an increasing number of engineering-intensive data science frameworks based on Machine Learning and Artificial Intelligence.
Ultimately, the future of data teams is one in which cross-functional collaboration becomes an essential part of the ideation and product creation processes.
End User Consumption
The true modern data analytics solutions go beyond raw computation powers and the ability to create machine learning models. It is accomplished by making use of these advanced algorithms to create a new user experience that makes accessing, consuming, and ultimately generating answers to the Big questions easier than ever before.
This user consumption layer of the stack can take various forms depending on the needs of the business. On one end is a familiar interface: the Visual Dashboard, but with significantly improved usability so that even inexperienced users can find the important details about their business.
Leverge your Biggest Asset Data
Inquire Now
Finally, what if the user is unsure of what to ask or how to determine what is interesting? It is critical in these scenarios to be able to detect irregularities – points of concern that a user would not think to ask or capture in a usual dashboard view.
Furthermore, the user should not have to ask anything explicitly. Instead, a one-time configuration in which the user informs the system about the types of information that are important to them should be sufficient for the system to proactively surface these anomalies and insights. A user might be concerned about changes in revenue, sales, costs, order volume by locality, department, or brand name. The user could be notified about these changes and why they are occurring on a regular or event-based basis.