- February 26, 2024
- Posted by: Indium
- Category: Data & Analytics
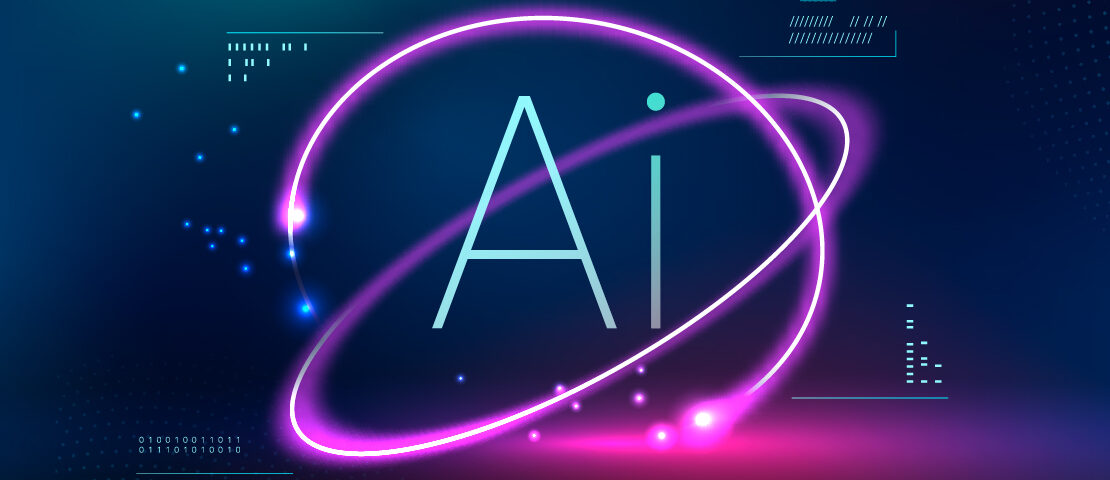
From planning travel itineraries to writing poetry, and even getting a research thesis generated, ChatGPT and its ‘brethren’ generative AI tools such as Sydney and Bard have been much in the news. Even generating new images and audio has become possible using this form of AI. McKinsey seems excited about this technology and believes it can provide businesses with a competitive advantage by enabling the design and development of new products and business process optimizations.
ChatGPT and similar tools are powered by generative artificial intelligence (AI), which facilitates the virtual creation of new content in any format – images, textual content, audio, video, code, and simulations. While the adoption of AI has been on the rise, Generative AI is expected to bring in another level of transformation, changing how we approach many business processes.
ChatGPT (generative pretrained transformer), for instance, was launched only in November 2022 by Open AI. But, from then to now, it has become very popular because it generates decent responses to almost any question. In fact, in just 5 days, more than a million users signed up. Its effectiveness in creating content is, of course, raising questions about the future of content creators!
Some of the most popular examples of Generative AI are images and chatbots that have helped the market grow by leaps and bounds. The generative AI market is estimated at USD 10.3 billion in 2022, and will grow at a CAGR of 32.2% to touch $53.9 billion by 2028.
Despite the hype and excitement around it, there are several unknown factors that pose a risk when using generative AI. For example, governance and ethics are some of the areas that need to be worked on due to the potential misuse of technology.
Check out this informative blog on deep fakes: Your voice or face can be changed or altered.
Decoding the secrets of Generative AI: Unveiling the learning process
Generative AI leverages a powerful technique called deep learning to unveil the intricate patterns hidden within vast data troves. This enables it to synthesize novel data that emulates human-crafted creations. The core of this process lies in artificial neural networks (ANNs) – complex algorithms inspired by the human brain’s structure and learning capabilities.
Imagine training a generative AI model on a massive dataset of musical compositions. Through deep learning, the ANN within the model meticulously analyzes the data, identifying recurring patterns in melody, rhythm, and harmony. Armed with this knowledge, the model can then extrapolate and generate entirely new musical pieces that adhere to the learned patterns, mimicking the style and characteristics of the training data. This iterative process of learning and generating refines the model’s abilities over time, leading to increasingly sophisticated and human-like outputs.
In essence, generative AI models are not simply copying existing data but learning the underlying rules and principles governing the data. This empowers them to combine and manipulate these elements creatively, resulting in novel and innovative creations. As these models accumulate data and experience through the generation process, their outputs become increasingly realistic and nuanced, blurring the lines between human and machine-generated content.
Evolution of Machine Learning & Artificial Intelligence
From the classical statistical techniques of the 18th century for small data sets, to developing predictive models, machine learning has come a long way. Today, machine learning tools are used to classify large volumes of complex data and to identify patterns. These data patterns are then used to develop models to create artificial intelligence solutions.
Initially, the learning models are trained by humans. This process is called supervised learning. Soon after, they evolve towards self-supervised learning, wherein they learn by themselves using predictive models. In other words, they become capable of imitating human intelligence, thus contributing to process automation and performing repetitive tasks.
Generative AI is one step ahead in this process, wherein machine learning algorithms can generate the image or textual description of anything based on the key terms. This is done by training the algorithms using massive volumes of calibrated combinations of data. For example, 45 terabytes of text data were used to train GPT-3, to make the AI tool seem ‘creative’ when generating responses.
The models also use random elements, thereby producing different outputs from the same input request, making it even more realistic. Bing Chat, Microsoft’s AI chatbot, for instance, became philosophical when a journalist fed it a series of questions and expressed a desire to have thoughts and feelings like a human!
Microsoft later clarified that when asked 15 or more questions, Bing could become unpredictable and inaccurate.
Here’s a glimpse into some of the leading generative AI tools available today:
ChatGPT: This OpenAI marvel is an AI language model capable of answering your questions and generating human-like responses based on text prompts.
DALL-E 3: Another OpenAI creation, DALL-E 3, possesses the remarkable ability to craft images and artwork from textual descriptions.
Google Gemini: Formerly known as Bard, this AI chatbot from Google is a direct competitor to ChatGPT. It leverages the PaLM large language model to answer questions and generate text based on your prompts.
Claude 2.1: Developed by Anthropic, Claude boasts a 200,000 token context window, allowing it to process and handle more data compared to its counterparts, as claimed by its creators.
Midjourney: This AI model, created by Midjourney Inc., interprets text prompts and transforms them into captivating images and artwork, similar to DALL-E’s capabilities.
Sora: This model creates realistic and imaginative scenes from text instructions. It can generate videos up to a minute long while maintaining visual quality and adherence to the user’s prompt.
GitHub Copilot: This AI-powered tool assists programmers by suggesting code completions within various development environments, streamlining the coding process.
Llama 2: Meta’s open-source large language model, Llama 2, empowers developers to create sophisticated conversational AI models for chatbots and virtual assistants, rivalling the capabilities of GPT-4.
Grok: Founded by Elon Musk after his departure from OpenAI, Grok is a new venture in the generative AI space. Its first model, Grok, known for its irreverent nature, was released in November 2023.
These are just a few examples of the diverse and rapidly evolving landscape of generative AI. As the technology progresses, we can expect even more innovative and powerful tools to emerge, further blurring the lines between human and machine creativity.
Underlying Technology
There are three techniques used in generative AI.
Generative Adversarial Networks (GANs)
GANs are powerful algorithms that have enabled AI to be creative by making two algorithms compete to achieve equilibrium.
Variational Auto-Encoders (VAE)
To enable the generation of new data, the autoencoder regularizes the distribution of encodings during training to ensure good properties of latent space. The term “variational” is derived from the close relationship between regularization and variational inference methods in statistics.
Transformers
A deep learning model, transformers use a self-attention mechanism to weigh the importance of each part of the input data differentially and are also used in natural language processing (NLP) and computer vision (CV).
Prior to ChatGPT, the world had already seen OpenAI’s GPT-3 and Google’s BERT, though they were not as much of a sensation as ChatGPT has been. Training models of this scale need deep pockets.
Generative AI Use Cases
Content writing has been one of the primary areas where ChatGPT has seen much use. It can write on any topic within minutes by pulling in inputs from a variety of online sources. Based on feedback, it can finetune the content. It is useful for technical writing, writing marketing content, and the like.
Generating images such as high-resolution medical images is another area where it can be used. Artwork can be created using AI for unique works, which are becoming popular. By extension, designing can also benefit from AI inputs.
Generative AI can also be used for creating training videos that can be generated without the need for permission from real people. This can accelerate content creation and lower the cost of production. This idea can also be extended to creating advertisements or other audio, video, or textual content.
Code generation is another area where generative AI tools have proved to be faster and more effective. Gamification for improving responsiveness and adaptive experiences is another potential area of use.
Governance and Ethics
The other side of the Generative AI coin is deep fake technology. If used maliciously, it can create quite a few legal and identity-related challenges. It can be used to implicate somebody wrongly or frame someone unless there are checks and balances that can help prevent such malicious misuse.
It is also not free of errors, as the media website CNET discovered. The financial articles written using generative AI had many factual mistakes.
OpenAI has already announced GPT4 but tech leaders such as Elon Musk and Steve Wozniak have asked for a pause in developing AI technology at such a fast pace without proper checks and balances. It also needs security to catch up and appropriate safety controls to prevent phishing, social engineering, and th generation of malicious code.
There is a counter-argument to this too which suggests that rather than pausing the development, the focus should be on developing a consensus on the parameters concerning AI development. Identifying risk controls and mitigation will be more meaningful.
Indeed, risk mitigation strategies will play a critical role in ensuring the safe and effective use of generative AI for genuine needs. Selecting the right kind of input data to train the models, free of toxicity and bias, will be important. Instead of providing off-the-shelf generative AI models, businesses can use an API approach to deliver containerized and specialized models. Customizing the data for specific purposes will also help improve control over the output. The involvement of human checks will continue to play an important role in ensuring the ethical use of generative AI models.
This is a promising technology that can simplify and improve several processes when used responsibly and with enough controls for risk management. It will be an interesting space to watch as new developments and use cases emerge.
To learn how we can help you employ cutting-edge tactics and create procedures that are powered by data and AI
Contact us
FAQ’s
Determining ownership of AI-generated content is a complex issue and ongoing legal debate. Here are some technical considerations:
(i). LLM architecture and licensing: The specific model’s architecture and licensing terms can influence ownership rights. Was the model trained on open-source data with permissive licenses, or is it proprietary?
(ii). Human contribution: If human intervention exists in the generation process (e.g., prompting, editing, curation), then authorship and ownership become more nuanced.
Several approaches can be implemented:
(i). Watermarking or fingerprinting techniques: Embedding traceable elements in generated content to identify the source and detect manipulations.
(ii). Deepfake detection models: Developing AI models specifically trained to identify and flag deepfake content with high accuracy.
(iii). Regulation and ethical frameworks: Implementing clear guidelines and regulations governing the development and use of generative AI, particularly for sensitive applications.
Neural networks are made up of interconnected nodes or neurons, organized in layers like the human brain. They form the backbone of Generative AI. They facilitate machine learning of complex structures, patterns, and dependencies in the input data to enable the creation of new content based on the input data.
Yes. In generative AI, machine learning happens without explicit labels or targets. The models capture the essential features and patterns in the input data to represent them in a lower-dimensional space.