- March 8, 2021
- Posted by: Abhimanyu Sundar
- Category: Data & Analytics
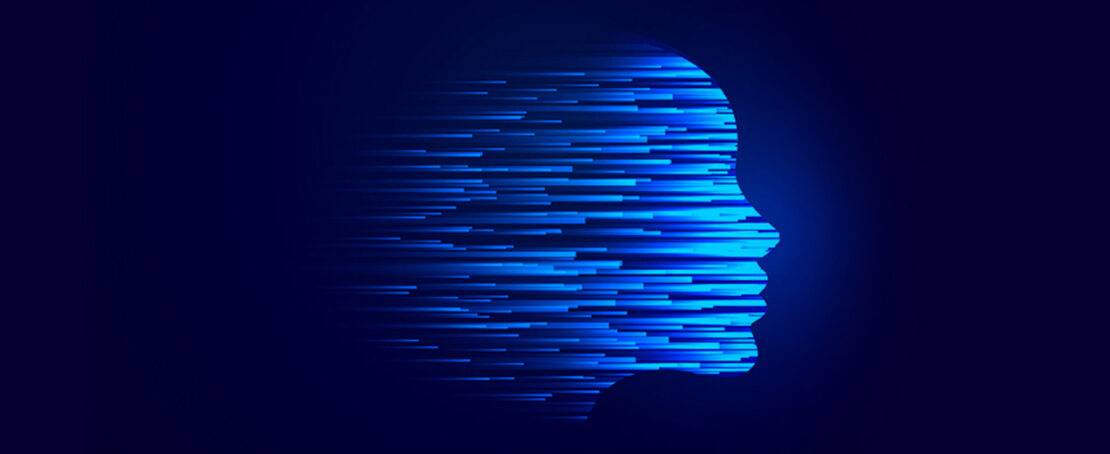
AI and Deep Learning
Much of today’s technological murmur and speculation revolves around artificial intelligence (AI) and deep learning.
The most talked about topic is whether artificial intelligence will replace manual labor in the years to come.
A very interesting statistic is that the number of jobs that require AI skills has grown by 450% from 2013 to present day.
It is known that AI is not exactly a new topic. However, with the rapid advancement in technology and accessibility to AI having increased tremendously, artificial intelligence has come to the fore.
To be very clear, we need to first understand that AI and deep learning are not the same thing and we need to understand how they are different.
Artificial intelligence is the simulation of intelligent human behavior by a computer system. This behavior entails a variety of tasks where human intelligence is required.
These tasks could range from language translations to decision making to visual pattern recognition to name a few.
Check out our Machine Learning and Deep Learning Services
Read More
On the other hand, deep learning takes inspiration from the knowledge of human brain biology to make use of artificial neural networks.
This leads to the emergence of algorithms that are extremely efficient at solving classification problems.
Deep learning can be categorized as an aspect of AI, which makes use of loads of precise data and complex neural networks to make machines learn thing in a way similar to humans.
These cutting edge technologies definitely have a few challenges that they face. Let’s see what these might be:
1. Requirement for Quality Data
Deep learning can function best when loads of quality data is available to it. As the data that is available grows, performance of the deep learning system also grows.
The time when a deep learning system can fail miserably is when quality data isn’t fed into the system.
Last year, Google deep learning systems were fooled by researchers. They altered the available data, in the sense; they added ‘noise’ to the already available data.
The errors weren’t high alert errors. They were errors where the system mistook rifles for turtles in the image recognition algorithms.
This further proves the point that for deep learning systems to be accurate, depend heavily on the right quantity and quality of data.
Noticing the fluctuation in results with a very small change in the input data further establishes the need more stability and accuracy in deep learning.
There may be domains like industrial applications where there is a lack of sufficient data. This limits the adoption of deep learning in such cases.
2. Bias problem of AI
The good or bad of an artificial intelligence system largely depends on the volume of data it is trained on. Therefore, it goes without saying that the future of AI systems depends on the volume and quality of data available to train them.
In reality, though, most of the data collected by organizations lacks quality and significance. They are firstly biased and largely only define the specifications and nature of a small demographic with interests such as gender, religion and the like.
What is the way forward?
The ideal scenario would be to build algorithms that can effectively track the problem of bias.
3. The AI Roll-out
Statistics this year show that 80% of the enterprise scale organizations are investing in AI.
This mounts a growing pressure on the organizations that develop AI technologies to move on from modeling and roll out production grade AI solution.
In order to consider the investment worthwhile, the AI solutions should be able to solve problems in the real world.
Operationalizing AI capabilities will be the key in the forthcoming years. Ensuring that the AI platforms are secure and are available easily will help deliver the required results in the required time frame.
In enterprises, AI should be able to help key stakeholders and executives make key decisions that may be strategic or tactical in nature.
4. Deep Learning is not Context Friendly
In deep learning, the ‘deep’ talks more about the architecture and not about the level of understanding that the algorithms are capable of producing.
Take the case of a video game. A deep learning algorithm can be trained to play Mortal Kombat really well and will even be able to defeat humans once the algorithm becomes very proficient.
Change the game to Tekken and the neural network will need to be trained all over again. This is because it does not understand the context.
With IoT devices making a huge impact and the need for real-time analysis being very important, the time taken to retrain deep learning models quickly will not be sufficient in order to keep up with the inflow of data.
5. Are Deep Learning Models Secure?
In the context of empowering cyber security, the applications of deep learning networks in that space are very exciting.
We need to keep in mind the change in output demonstrated by deep learning models due to a slight data input modification.
This may also leave the door open to malicious attacks.
We now have cars that partially run on deep learning models.
Suppose someone accessed the model and altered the input data, the vehicle could behave in unexpected ways and this could prove to be dangerous.
There have been cases reported about the same.
Is Your Application Secure? We’re here to help. Talk to our experts Now
Inquire Now
To Summarize
AI and Deep Learning have massive potential and their applications are second to none. Along with all the buzz and excitement around these technologies, there are also few challenges.
These challenges however will definitely be overcome in the forthcoming years.
Developers and data scientists work around the clock in all parts of the world figuring out how these challenges can be made a thing of the past.
While investing in technologies like AI and Deep Learning, it is always good to know what challenges you may face with the technologies.