- October 6, 2023
- Posted by: Indium
- Category: Data & Analytics
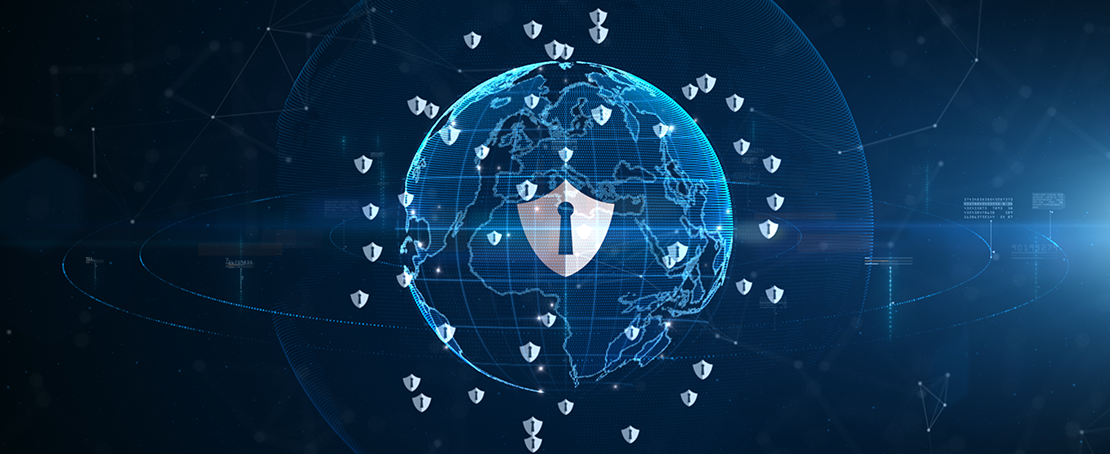
Cybersecurity has always been a growing cause of concern for businesses worldwide. Every day, we hear stories of cyberattacks on various organizations, leading to heavy financial and data losses. For instance, in May 2023, T-Mobile announced its second data breach, revealing the PINs, full names, and phone numbers of over 836 customers. This was not an isolated incident for the company; earlier in January 2023, T-Mobile had another breach affecting over 37 million customers. Such high-profile breaches underscore the vulnerabilities even large corporations face in the digital age.
According to Cybersecurity Ventures, it is estimated that the global annual cost of cybercrime is predicted to reach $8 trillion USD in 2023. Additionally, the damage costs from cybercrime are anticipated to soar to $10.5 trillion by 2025. The magnitude of these attacks emphasizes the critical need for organizations to prioritize cybersecurity measures and remain vigilant against potential threats.
While cyber threats continue to evolve, technology consistently showcases its capability to outsmart them. Advanced AI systems proactively detect threats, and quantum cryptography introduces near-unbreakable encryption. Behavioral analytics tools, like Darktrace, pinpoint irregularities in network traffic, while honeypots serve as decoys to lure and study attackers. A vigilant researcher’s swift halting of the WannaCry ransomware’s spread exemplifies technology’s edge. These instances collectively underscore technology’s potential for countering sophisticated cyber threats.
Generative AI (GenAI) is revolutionizing cybersecurity with its advanced machine learning algorithms. GenAI identifies anomalies that often signal potential threats by continuously analyzing network traffic patterns. This early detection allows organizations to respond swiftly, minimizing potential damage. GenAI’s proactive and adaptive approach is becoming indispensable as cyber threats grow in sophistication, with its market valuation projected to reach USD 11.2 billion, with a CAGR of 22.1% by 2032, reflecting its rising significance in digital defense strategies.
Decoding the GenAI mechanism
The rapid evolution of Generative AI, especially with the advent of Generative Adversarial Networks (GANs), highlights the transformative power of technology. Companies, including NVIDIA, have successfully leveraged GenAI for security, using it to detect anomalies and enhance cybersecurity measures. Since its inception in the 1960s, GenAI has transitioned from basic data mimicry to creating intricate, realistic outputs. Presently, an impressive 81% of companies utilize GenAI for security. Its applications span diverse sectors, offering solutions that were once considered the realm of science fiction. NVIDIA’s success story is a testament to the relentless pursuit of innovation and the boundless possibilities of AI.
GenAI performs data aggregation to identify security threats and take the necessary actions to maintain data compliance across your organization. It collects data from diverse sources, using algorithms to spot security anomalies. Upon detection, it alerts administrators, isolates affected systems or blocks malicious entities. Ensuring data compliance, GenAI encrypts sensitive information, manages access, and conducts audits. According to projections, by 2025, GenAI will synthetically generate 10% of all test data for consumers dealing with use cases. Concurrently Generative AI systems like ChatGPT and DALL-E 2 are making waves globally. ChatGPT acts as a virtual tutor in Africa and bolsters e-commerce in Asia, while DALL-E 2 reshapes art in South America and redefines fashion in Australia. These AI systems are reshaping industries, influencing how we learn, create and conduct business.
Generative AI, through continuous monitoring and data synthesis, provides real-time security alerts, ensuring swift threat detection and response. This AI capability consolidates diverse data into a centralized dashboard, offering decision-makers a comprehensive view of operations. Analyzing patterns offers insights into workflow efficiencies and potential bottlenecks, enhancing operational visibility. In 2022, around 74% of Asia-Pacific respondents perceived security breaches as significant threats. With Generative AI’s predictive analysis and trend identification, businesses can anticipate challenges, optimize operations, and bolster security.
Tomer Weingarten, the co-founder and CEO of SentinelOne, a leading cybersecurity company, said, “Generative AI can help tackle the biggest problem in cybersecurity now:” With GenAI, complex cybersecurity solutions can be simplified to yield positive outcomes.
The role of Generative AI in cybersecurity risk mitigation
Reuben Maher, the Chief Operating Officer of Skybrid Solutions, who oversees strategic initiatives and has a deep understanding of the intricacies of modern enterprise challenges, stated, “The convergence of open-source code and robust generative AI capabilities has powerful potential in the enterprise cybersecurity domain to provide organizations with strong and increasingly intelligent defenses against evolving threats.”
There are many open source (Llama2, MPT, Falcon etc.) and paid (ChatGPT, PALM, Claude etc.) which can be used based on the available infrastructure and complexity of the problem.
Fine-tuning is a technique in which pre-trained models are customized to perform specific tasks involving taking an existing model that has already been trained and adapting it to a narrower subject or a more focused goal.
It involves three key steps:
1. Dataset Preparation: Gather a dataset specifically curated for the desired task or domain.
2. Training the Model: Using the curated dataset, the pre-trained model is further trained on the task-specific data. The model’s parameters are adjusted to adapt it to the new domain, enabling it to generate more accurate and contextually relevant responses.
3. Evaluation and Iteration: Once the fine-tuning process is complete, the model is evaluated using a validation set to ensure it meets the desired performance criteria. If necessary, the process can be iterated by the model again with adjusted parameters to improve performance further.
Use case: using Generative AI models trained on open-source datasets of known cyber threats, organizations can simulate various attack scenarios on their systems. This “red teaming” approach aids in identifying vulnerabilities before actual attackers exploit them.
Proactive defense with Generative AI
Generative AI revolutionizes cybersecurity by enabling proactive defense strategies in the face of a rapidly evolving threat landscape. Through the application of Generative AI, organizations can bolster their security posture in multiple ways. First and foremost, Generative AI facilitates robust threat modeling, allowing organizations to identify vulnerabilities and potential attack vectors within their systems and networks. Furthermore, it empowers the simulation of complex cyber-attack scenarios, enabling security teams to understand how adversaries might exploit these vulnerabilities. In addition, Generative AI’s continuous analysis of network behaviors detects anomalies and deviations from established patterns, providing real-time threat detection and response capabilities. Perhaps most crucially, it excels in predicting potential cyber threats by leveraging its ability to recognize emerging patterns and trends, allowing organizations to proactively mitigate risks before they materialize. In essence, Generative AI serves as a unified and transformative solution that empowers organizations to anticipate, simulate, analyze, and predict cyber threats, ushering in a new era of proactive cybersecurity defense.
Enhanced anomaly detection:
Generative AI is renowned for recognizing patterns. Analyzing historical data through autoencoders to understand the intricate patterns establishes a baseline of a system’s “normal” behavior. When it detects deviations, such as unexpected data spikes during off-hours, it flags them as anomalies. This deep learning-driven approach surpasses conventional methods, enabling Generative AI to identify subtle threats that might elude traditional systems, making it an invaluable asset in cybersecurity.
Enhanced training in data generation
Generative AI can excel at producing synthetic data, especially images, by discerning patterns within the datasets. This unique ability enriches training sets for machine learning models, ensuring diversity and realism. It aids in data augmentation and ensures privacy by creating non-identifiable images. Whether tabular data, time series, or even intricate formats such as images and videos, Generative AI guarantees that the training data is comprehensive and mirrors real-world scenarios.
Simulating cyberattack scenarios:
In the realm of cybersecurity, the utility of Generative AI in accurately replicating training data is indeed paramount when simulating cyberattacks scenarios. This unique capability enables organizations to adopt a proactive stance by recognizing and mitigating potential threats before they escalate. Let’s delve deeper into the technical aspects, particularly addressing the challenge of dealing with highly imbalanced datasets:
Accurate Data Replication and Simulation:
Generative AI models, such as Generative Adversarial Networks (GANs) or Variational Autoencoders (VAEs), excel at replicating training data accurately. Here’s how they can be applied in a cybersecurity context:
1. GANs for Data Generation: GANs consist of a generator and a discriminator. The generator learns to generate data samples that are indistinguishable from real data, while the discriminator tries to tell real data from generated data. In cybersecurity, GANs can be trained on historical data to accurately replicate various network behaviors, traffic patterns, and system activities.
2. Variational Autoencoders (VAEs): VAEs are probabilistic generative models that learn the underlying structure of data. They can be used to generate synthetic data points that closely resemble the training data while capturing its distribution. VAEs can be particularly useful for simulating rare but critical events that may occur during cyberattacks.
3. Large Language Models (LLMs): LLMs, such as GPT-4, can be harnessed for text-based data generation and enrichment. They excel in generating natural language descriptions of cybersecurity events, threat scenarios, and incident reports. This text data can augment the output of GANs and VAEs, providing additional context and narrative to the simulated data, making it more realistic and informative.
Handling Imbalanced Datasets:
Cybersecurity datasets are often highly imbalanced, with a vast majority of data points representing normal behavior and only a small fraction indicating cyber threats. Generative AI can help mitigate this issue:1.
1. Oversampling Minority Class: Generative AI can generate synthetic examples of the minority class (cyber threats) to balance the dataset. This ensures that the model is not biased towards the majority class (normal behavior).
2. Anomaly Generation: Generative AI can be fine-tuned to generate data points that resemble anomalies or rare events. This helps in simulating cyber threats effectively, even when they are infrequent in the training data.
Innovative security tool development
Generative AI can be used to devise new security tools. From generating phishing emails to counterfeit websites, harnessing this technology empowers security analysts in threat simulation, training enhancement, proactive defense and more to proactively identify potential threats and stay proactive toward ever-changing cyber challenges. However, while its potential is vast, ethical concerns arise as malevolent actors could misuse Generative AI for malicious intent. It’s imperative to establish stringent guidelines and controls to prevent such misuse.
Automated incident response and remediation:
Generative AI-driven systems offer the potential for rapid response and enhanced protection in cybersecurity by leveraging advanced algorithms to analyze and respond to threats efficiently. Here, we’ll dive into more technical details while addressing the associated challenges:
Swift Attack Analysis and Response:
Generative AI-driven systems utilize advanced machine learning and deep learning algorithms for swift attack analysis. When a potential threat is detected, these systems employ techniques such as:
- 1. Behavioral Analysis: Continuously monitoring and analyzing network and system behavior patterns to detect anomalies or suspicious activities indicative of an attack.
- 2. Pattern Recognition: Leveraging pattern recognition algorithms to identify known attack signatures or deviations from normal behavior.
- 3. Predictive Analytics: Employing predictive models to forecast potential threats based on historical data and real-time information.
- 4. Threat Intelligence Integration: Integrating real-time threat intelligence feeds to stay updated on the latest attack vectors and tactics used by malicious actors.
Challenges and Technical Details:
- 1. False Positives:
– Addressing false positives involves refining the machine learning models through techniques like feature engineering, hyperparameter tuning, and adjusting the decision thresholds.
– Employing ensemble methods or anomaly detection algorithms can help reduce false alarms and improve the accuracy of threat detection.
- 2. Adversarial Attacks:
– To mitigate adversarial attacks, Generative AI models can be hardened by implementing techniques such as adversarial training and robust model architectures.
– Regularly retraining models with updated data and re-evaluating their security can help in detecting and countering adversarial attempts.
- 3. Complexity:
– To make AI models more interpretable, techniques such as model explainability and feature importance analysis can be applied. This helps in understanding why a particular decision or classification was made.
– Utilizing simpler model architectures or incorporating rule-based systems alongside AI can provide transparency in decision-making.
- 4. Over-Reliance:
– Human experts should always maintain an active role in cybersecurity. AI-driven systems should be viewed as aids rather than replacements for human judgment.
– Continuous training and collaboration between AI systems and human experts can help strike a balance between automation and human oversight.
By effectively addressing these challenges and leveraging the technical capabilities of Generative AI, cybersecurity systems can rapidly identify, understand, and respond to cyber threats while maintaining a balance between automation and human expertise.
Navigating GenAI: Meeting complex challenges with precision
Generative AI presents a transformative world, but it is not without obstacles. Success lies in the meticulous handling of the complex challenges that arise. Explore the crucial hurdles that must be addressed responsibly and effectively to realize the potential of GenAI.
1.Data management
LLM’s pioneers of AI Evolution: Large Language Models (LLMs) are crucial for AI advancements, significantly enhancing the capabilities of artificial intelligence and paving the way for more sophisticated applications and solutions.
Third-party risks: The storage and utilization of this data by third-party AI providers can expose your organization to unauthorized access, data loss, and compliance issues. Establishing proper controls and comprehensively grasping the data processor and data controller dynamics is crucial to mitigating the risks.
2. Amplified threat landscape
Sophisticated phishing: The emergence of sophisticated phishing techniques has lowered the threshold for cybercriminals. These include deep fake videos or audio, customized chat lures, and highly realistic email duplications, which are on the rise.
Examples include CEO fraud, tax scams, COVID-19 vaccine lures, package delivery notifications, and bank verification messages designed to deceive and exploit users.
Insider threats: By exploiting GenAI, insiders with in-depth knowledge of their organization can effortlessly create deceptive and fraudulent content. The potential consequences of an insider threat involve the loss of confidential information, data manipulation, erosion of trust, and legal and regulatory repercussions. To counteract these evolving threats, organizations must adopt a multi-faceted cybersecurity approach, emphasizing continuous monitoring, employee training, and the integration of advanced threat detection tools.
3. Regulatory and legal hurdles
Dynamic compliance needs: In the ever-evolving GenAI landscape, developers and legal/compliance officers must continually adapt to the latest regulations and compliance studies. Staying abreast of new regulations and stricter enforcement of existing laws is crucial to ensuring compliance.
Exposure to legal risks: Inadequate data security measures can result in the disclosure of valuable trade secrets, proprietary information, and customer data, which can have severe legal consequences and negatively impact a company’s reputation.
For instance, recently, the European Union’s GDPR updates emphasized stricter consent requirements for AI-driven data processing, impacting GenAI developers and compelling legal teams to revisit compliance strategies.
Organizations should prioritize continuous training, engage regulatory consultants, leverage compliance software, stay updated with industry best practices, and foster open communication between legal and tech teams to combat this.
4. Opaque model
Black-box dilemma: Generative AI models, especially deep learning ones, are often opaque. It is difficult for cybersecurity experts and business leaders to trust and validate their outputs because of their high accuracy and lack of transparency in decision-making. To enhance trust and transparency, organizations can adopt Explainable AI (XAI) techniques, which aim to make the decision-making processes of AI models more interpretable and understandable.
Regulatory and compliance challenges: In sectors like finance and healthcare, where explainability is paramount, AI’s inability to justify its decisions can pose regulatory issues. Providing clear reasons for AI-driven decisions, such as loan denials or medical claim rejections. To address this, organizations can implement auditing and validation frameworks that rigorously test and validate AI decisions against predefined criteria, ensuring consistency and accountability.
Undetected biases: The inherent opaqueness of these models can conceal biases in data or decision-making. These biases might remain hidden without transparency, leading to potentially unfair or discriminatory results. In response, it’s essential to implement rigorous testing and validation processes, utilizing tools and methodologies specifically designed to uncover and rectify hidden biases in AI systems.
Troubleshooting difficulties: The lack of clarity in generative AI models complicates troubleshooting. Pinpointing the cause of errors becomes a formidable task, risking extended downtimes and potential financial and reputational repercussions. To mitigate these challenges, adopting a layered diagnostic approach combined with continuous monitoring and feedback mechanisms can enhance error detection and resolution in complex AI systems.
5. Technological adaptation
Rapid tool emergence: The unexpected rise of advanced GenAI tools like ChatGPT, Bard, and GitHub Copilot has caught enterprise IT leaders off guard. To tackle the challenges posed by these tools, implementing Generative AI Protection solutions is absolutely essential. To effectively integrate these solutions, organizations should prioritize continuous training for IT teams, fostering collaboration between AI experts and IT personnel, and regularly updating security protocols in line with the latest GenAI advancements.
Enterprises can rely on Symantec DLP Cloud and Adaptive Protection to safeguard their operations against potential attacks. These innovative solutions offer comprehensive capabilities to discover, monitor, control, and prioritize incidents. To harness the full potential of these solutions, enterprises should integrate them into their existing IT structure, conduct regular system audits, and ensure that staff are trained on the latest security best practices and tool functionalities.
Discover how Indium Software can empower organizations with Generative AI
Indium Software empowers organizations to seamlessly integrate AI-driven systems into their workplace environments, addressing comprehensive security concerns. By harnessing the prowess of GenAI, the experts at Indium Software deliver diverse solutions that elevate and streamline business workflows, leading to tangible and long-term gains.
In addition to these, the AI experts at Indium Software offer a wide range of services. These include GenAI strategy consulting, end-to-end LLm/GenAI product development, GenAI model pre-training, model fine-tuning, prompt engineering, and more.
Conclusion
In the cybersecurity landscape, Generative AI emerges as a game-changer, offering robust defenses against sophisticated threats. As cyber challenges amplify, Indium Software’s pioneering approach in harnessing GenAI’s capabilities showcases the future of digital protection. For businesses, embracing such innovations is no longer optional—survival and growth must stay ahead in this competitive digital era and safeguard their valuable assets.